In the previous post, Think You’re Intelligent? You Ain’t seen nothin’ Yet: Part I, (https://www.intelligence-and-iq.com/think-youre-intelligent-you-aint-seen-nothin-yet-part-i/) we traced the early stages of artificial intelligence (AI) en route to a discussion of the latest AI software ChatGPT (Generative, Pre-trained Generator) that is rocking the intellectual world. In that post we visited the defeat of chess master Gary Kasparov in what was billed as “the shootout between carbon-based and silicon-based intelligence.” In that 1997 contest, it became evident that artificial intelligence was moving ahead of human intelligence in the domain of strategy games. Was this merely the conquest in a small domain of intellectual skill or was it a harbinger of an expanding reach of the silicon-based intelligence?
In this post, we visit the next stage in the encroachment of silicon-based intelligence on human cognition, excerpted from the book, Intelligence: Where we Were, Where we Are, & Where we’re Going. https://www.intelligence-and-iq.com/intelligence/.
In the aftermath of the Deep Blue victory, there was a denial of the closing gap between human and machine intelligence, asserting that in order to prove that artificial intelligence is “real intelligence” it would have to outperform humans in other realms.
During the second half of the 20th century, IQ tests provided the prime measure of intelligence, so the Turing test would certainly require computers to demonstrate competence on such tests in order to qualify as “intelligent.” This presented a challenge of much greater magnitude than chess, because speed in both computation and deductive processes are tasks for which the computer is ideally suited, while human intelligence evolves through induction, i.e., synthesizing millions of observations into general understandings.
Interpreting Natural Language
The Miller Analogies tests published by Pearson Education are widely used for graduate school admissions in the United States, because they are strongly correlated with student academic performance. A sample item from one of these tests is:
(____) is to INNOCUOUS as REPREHENSIBLE is to PRAISEWORTHY
A. pretentious B. virulent. C. antiseptic. D. widespread
To answer this question correctly, it is insufficient to merely know the meanings of the words, but it is also necessary to understand their relationships to one another. Since “reprehensible” and “praiseworthy” are polar opposites, an intelligent being would seek the polar opposite of “innocuous” and select the answer “virulent.” This prompts us to investigate how humans acquire concepts and learn the relationships expressed in language.
During the formative years, the young child acquires language through the dual processes of assimilation and accommodation (described in chapter 6). Wittgenstein, Piaget, and others proposed that “meaning” derives from a synthesis of these experiences into relationships that consolidate as concepts or ideas. For example, a child understands that if Zelda is Alpha’s daughter, then Alpha is Zelda’s mother. However, a computer requires that this information be pre-programmed. For humans, the millions of connections established in various contexts throughout life become embedded in our understanding of language. For example, the sentence, “Time flies like an arrow; fruit flies like a banana,” requires a sophisticated understanding of language that few computers in the 1980’s and 1990’s could process. From experience, humans realize that in the first clause, “flies” is used as a verb with “like” as a preposition introducing a simile, while in the second clause, “flies” is used as a noun with “like” as a verb meaning “like to eat.” It takes context to understand that bananas (usually) don’t fly. Indeed, an understanding of metaphor requires rich experiences in various contexts that enable the identification of parallel structures in similar situations. For example, a savings and loan commercial offering home improvement funds to repaint a house used the tag line, “You choose the color, and we’ll supply the green.” Most humans would understand that “green” was a metaphor for money, but in order for a computer to successfully interpret the intent of the ad, it would need to have this information in advance.
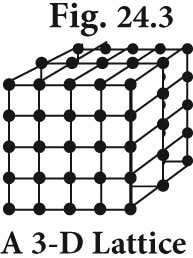
One computer programming technique that associated words of similar meaning or context involved the creation of an n-dimensional lattice that located words at the intersection of lattice lines connecting semantically related concepts. In the example above, the phrase “fruit flies” might reside at the intersection of a line listing every kind of fruit with another line listing things that fly. These lattices linking words that may occur in related contexts were called “associative nets.” However, the number of ways that words can be combined in pairs and in related contexts presented an overwhelming programming challenge. Developing a computer that could interpret natural language as well as understand metaphor would prove to be an even greater challenge than creating a chess-playing machine.
In the unrelenting march to simulate human intelligence and overtake it in capability, there was still a way to go, but the as march continued the gap between carbon-based and silicon-based intelligence continued to narrow. We’ll examine the next stage in Think You’re Intelligent? You Ain’t Seen Nothin’ Yet!: Part III.